In this blog post we define the concept of functional data analysis.
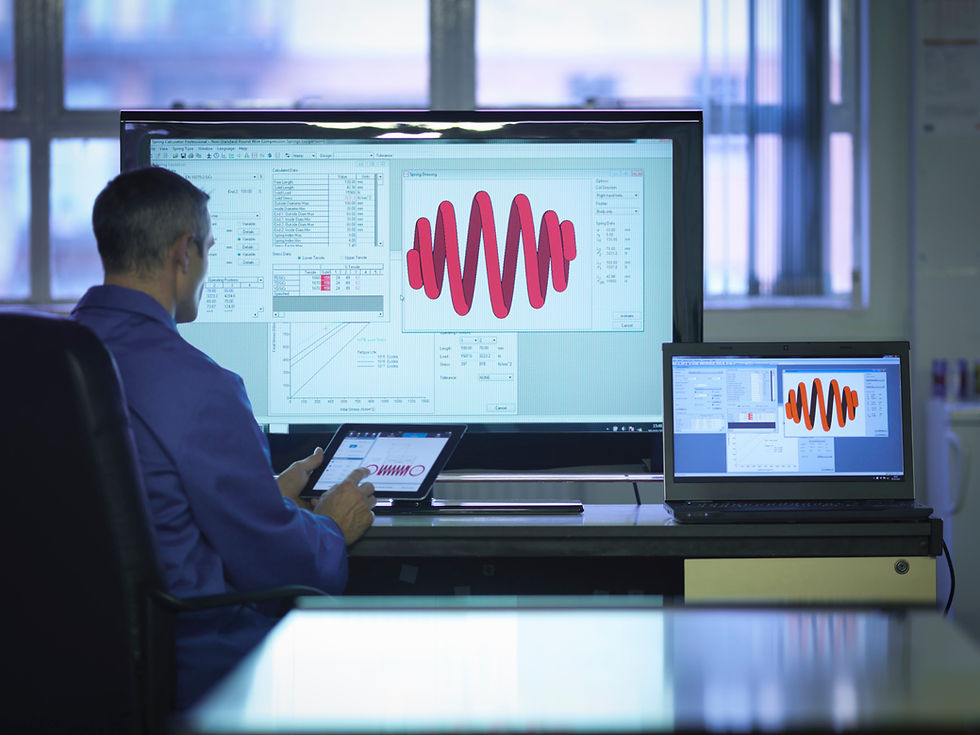
With recent data storage developments, real-time data are now available in many fields. Researchers, companies, and data analysis enthusiasts are trying to extract new insight from this rich information. Like any other big data technique, functional data analysis (FDA) has gained in popularity during the last decade.
In this blog post, I will introduce a series on FDA. This first episode is presented as follows. The first section gives a simple definition of functional data. Section 2 presents the benefits of using this data configuration with an example given for illustration.
1. Definition
Functional data analysis is a field of Statistical analysis that manipulates curves or functions, surfaces and volumes.
Usually, in the standard data analysis context, we have a sample of data that is a collection of numbers or scalars following a certain distribution. In contrast, in the framework of functional data analysis, the sample considered is a collection of functions following the same pattern. This field is not that new. It was introduced since 1950 by Grenander and Rao (1958). This concept is widely discussed by Ramsay (1982) and Ramsay & Dalzell (1991). A nice book to get introduced by this idea is the handbook by Ramsay & Silverman (2005).
2. Why using Functional Data analysis?
There are several reasons why should or we can consider functional data analysis. For the sake of this blog post, I will present 3 situations.
Interpretability purpose
We can be interested in presenting and interpreting results in a certain way. A simple example could be observed in climate analysis. Indeed, a company in the electricity industry can be interested in analyzing the relationship between temperature and precipitations and make possible the results being displayed in real-time in Canada. The following figure presents the temperature and precipitations of 35 weather stations in Canada. Then, each weather station is considered as an observation represented by a curve of temperature and a curve of precipitations. The temperature and precipitations are observed in a certain period of the day.
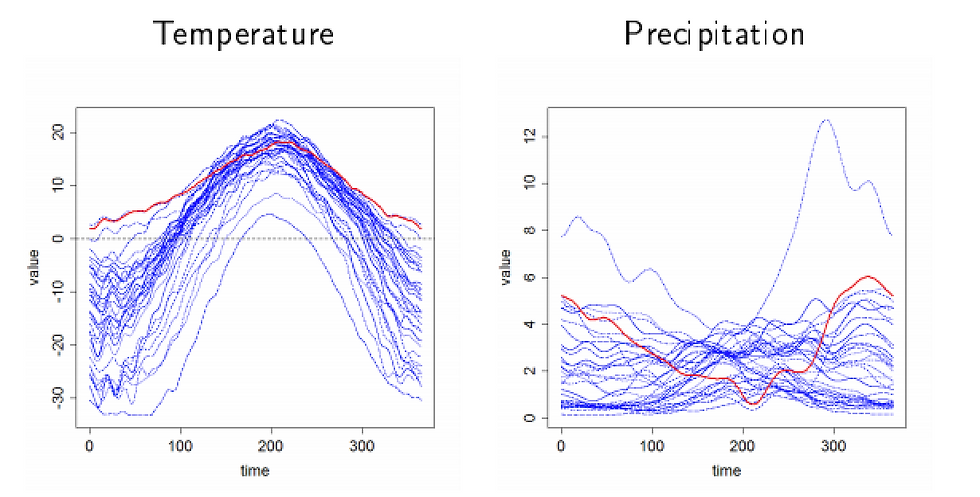
Improve the forecasts
In the context of forecasting in Finance using classical time series models, let us consider that we consider that we have apple stock price displayed on daily frequency. In this context, each daily value is a number or a scalar and we can use a variant of an autoregressive model for forecasting purposes.
But using this approach, we ignore the whole dynamics of apple stock within a trading day. This additional information is useful to improve the forecasts. Then in the functional time series model, we can consider that each day is a curve or a collection of 390 apple price values, each representing a 1-minute display. Therefore one can still use an autoregressive model where each observation is a function. The following graph gives a representation of the collection of predictors and responses functions on the period considered.
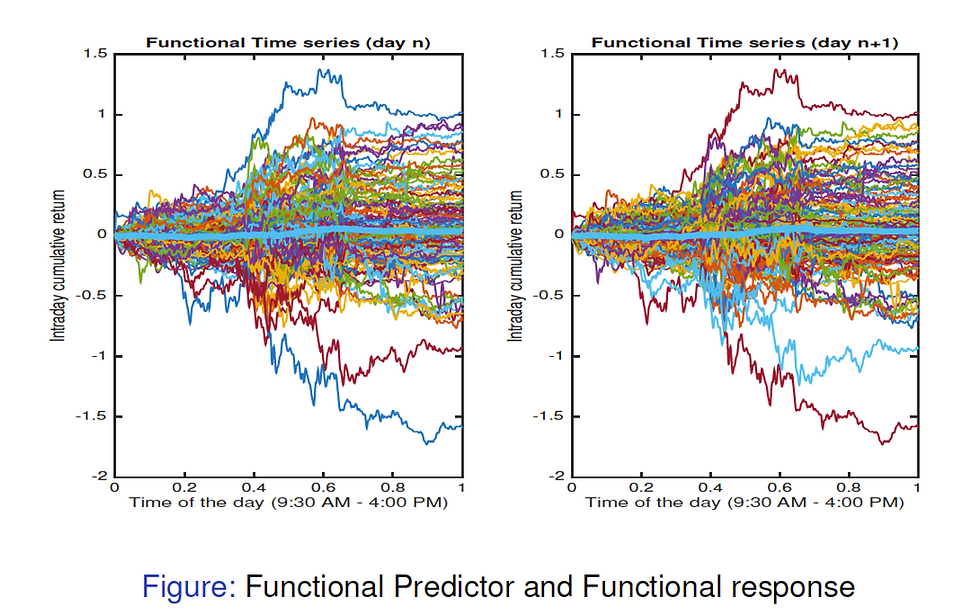
Follow a theoretical concept
In Finance, the yield bond is an important variable used for option pricing and sentiment analysis. In theoretical results, this variable is usually considered as a function of a continuum of time to maturity but in practice, the values of this variable are only observed for a few numbers of time to maturity (See Diebold & Li (2005)). In fact, this variable can be smoothed in order to transform the original variable into a function of a continuum of time to maturity.
In the next post, we will see some real applications of the functional data analysis.
Feel free to comment or ask questions about the topic.
Comentários